OK Computer: How computers will—and won't—change the NFL

In 1996, IBM’s Deep Blue became the first supercomputer to defeat a chess grandmaster, Garry Kasparov, in a game. A year later Deep Blue edged Kasparov 3 1/2–2 1/2 in a full match. Why should you, a football fan, care? Because, as the late linebacker Junior Seau once said, “football is a chess game.”
Deep Blue defeated Kasparov by brute force, scanning through 200 million moves per second. And, ominously, over the last two decades that computational force has only gotten more brutal. At chess tournaments played in Bilbao, Spain, in 2004 and ’05, a team of three computers defeated their human opponents 8½–3½ and 8–4, respectively. But that was two decades ago. modern smartphones make even Deep Blue look painfully slow: A Samsung Galaxy S5, for example, can perform 140 billion floating-point operations per second, more than 10 times the speed of IBM’s old machine. Moore’s Law predicts that computational power doubles roughly every two years, so by Super Bowl 100, in 2066, computers should be several million times faster than today. Imagine a robot Bill Belichick flicking through a digital playbook of trillions of moves during the 40-second gap between plays.
The BCS computers already made their mark in the college game, before a human-only playoff committee overthrew them last year. The computers were either a digital force for good or evil, depending on whether they raised or lowered your school’s ranking. A company called Edge Up Sports is using Watson, IBM’s cognitive computing system, to gain an edge in fantasy football. Jim Rushton, head of IBM’s Sports & Entertainment division, predicts that in the next few years Watson could help teams predict and reduce injuries, and pick the best players from the draft.
Look for new entries in the Super Bowl 100 series, presented by Gatorade and Microsoft Surface, at SI.com/SB100 and Wired.com/SB100
Chapter 1, Oct. 7 TRAINING
Chapter 2, Oct. 28 EQUIPMENT
Chapter 3, Nov. 18 STADIUMS
Chapter 4, Dec. 9 CONCUSSIONS
Chapter 5, Dec. 16 MEDIA
Chapter 6, Dec. 30 VR
Chapter 7, Jan. 6 NFL IN SOCIETY
Chapter 8, Jan. 13 TRACKING
Chapter 9, Jan. 20 STRATEGY
Chapter 10, Jan. 27 SB 100
ROAD MAP TO
THE FUTURE
The application of artificial intelligence to play-calling is already upon us. Last spring, two students at North Carolina State, William Burton and Michael Dickey, built a model to predict whether an NFL team would pass or run, information that would hugely benefit defensive coordinators Tested against decisions made in a 2014 Cowboys-Jaguars game, the model picked the play type 91.6% of the time. Before this season SI approached a big-data analysis company, Splunk, and asked whether it could forecast the next play. Nate McKervey, Splunk’s director of technical marketing, plugged 15 years’ worth of NFL data into his company’s platform. On Nov. 8, with the Falcons at their own 46-yard line with 5:21 to go in the third quarter, McKervey’s friend asked him to make a prediction. McKervey glanced at his phone. It showed that Atlanta would line up in shotgun formation, and quarterback Matt Ryan would pass short left. Although the throw was incomplete, everything matched perfectly.
Football, of course, is more complicated than pass-versus-run. The number of possible scenarios on a field far outnumber the options on a chess board: Players aren’t confined to squares, and the pawns along the line of scrimmage or the rooks split wide come in a variety of sizes. The fact that calls must be made within a 40-second window further raises the computational challenge. “Things aren’t yet real-time,” says Stefan Groschupf, CEO and co-founder of Datameer, a big-data analytics company. “You can compute historical data and then you can make recommendations,” but you can’t do that fast enough to make game-time decisions. Yet. But two key factors might help football develop that sort of computational ability, says IBM’s Rushton: “a tremendous amount of data and a tremendous amount of money.”
The two AI research areas with the most potential relevance for football are machine learning and game theory. Machine learning, says Yoav Shoham, a professor emeritus of computer science at Stanford and a principal scientist at Google, is “Moneyball on steroids.” It’s how Watson does what it does, and how Google’s Artificial Brain learned to identify cats in YouTube videos in 2012. Given a large set of data, a clever computer can search for patterns and learn from past actions and outcomes. If everyone always punts on fourth-and-long, the computer will always punt on fourth-and-long.
The power of this approach lies in the size of the data set—the more information available, the smarter the computer. “You could go down and model the physiology of each player,” says Shoham. “You could model their mental state, what they had for breakfast.” But data gaps can be a problem. And teams keep secrets from one another. Most practices and training camps are off-limits to the media for fear of giving away sensitive information to opposing teams. One way to fill in holes, of course, is espionage. The Patriots were found guilty of videotaping Jets’ defensive signals back in 2007, but technological advances already make that attempt seem clumsy and outdated. Take Eulerian Video Magnification: Developed by researchers at MIT, this process can magnify color changes and movement in any video feed. EVM can be used to measure a person’s heart rate—through changes in skin tone—and breathing pattern from afar, or even to reproduce someone’s voice from the vibrations the sound induces in a nearby object. Taken to its football extreme, a team could run EVM on the broadcast feed of a game to deduce which players in an opponent’s line are getting tired, or to lip-read an opposing coach regardless of whether he might be holding a clipboard over his mouth.
Game theory, in contrast, works best when there are knowledge gaps. Made famous by the 2001 film A Beautiful Mind, which was inspired by the life of game-theorist John Nash, game theory can guide teams on how to act in situations where they don’t know for sure how their opponents will behave. According to Matthew O. Jackson, a professor of economics at Stanford who teaches an online game theory course with Shoham, last year’s Super Bowl was a perfect example. “If you’re the Seahawks, you can’t just always give it to [Marshawn] Lynch because the other team can just key off that,” Jackson says. “And if you’re the Patriots, you can’t just assume [Seattle is] going to give it to Lynch.” Sometimes unexpectedly playing the weaker of two options is better—such as going for it on fourth-and-long.
But while machine learning might fine-tune scouting reports, and game theory might offer situational options, both are ultimately just tools for a coach to employ. And in 2066, that coach will still be human. Probably.
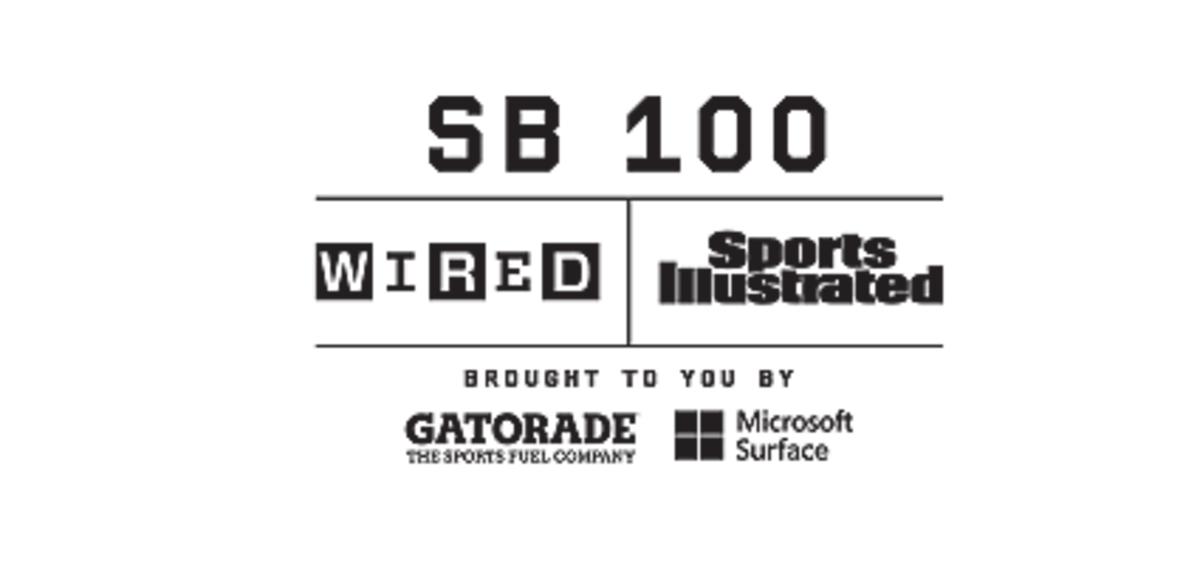